March 15, 2021
Racism In, Racism Out: AI Reproduces Healthcare Inequity
By Timothy LaRock and Benjamin Batorsky
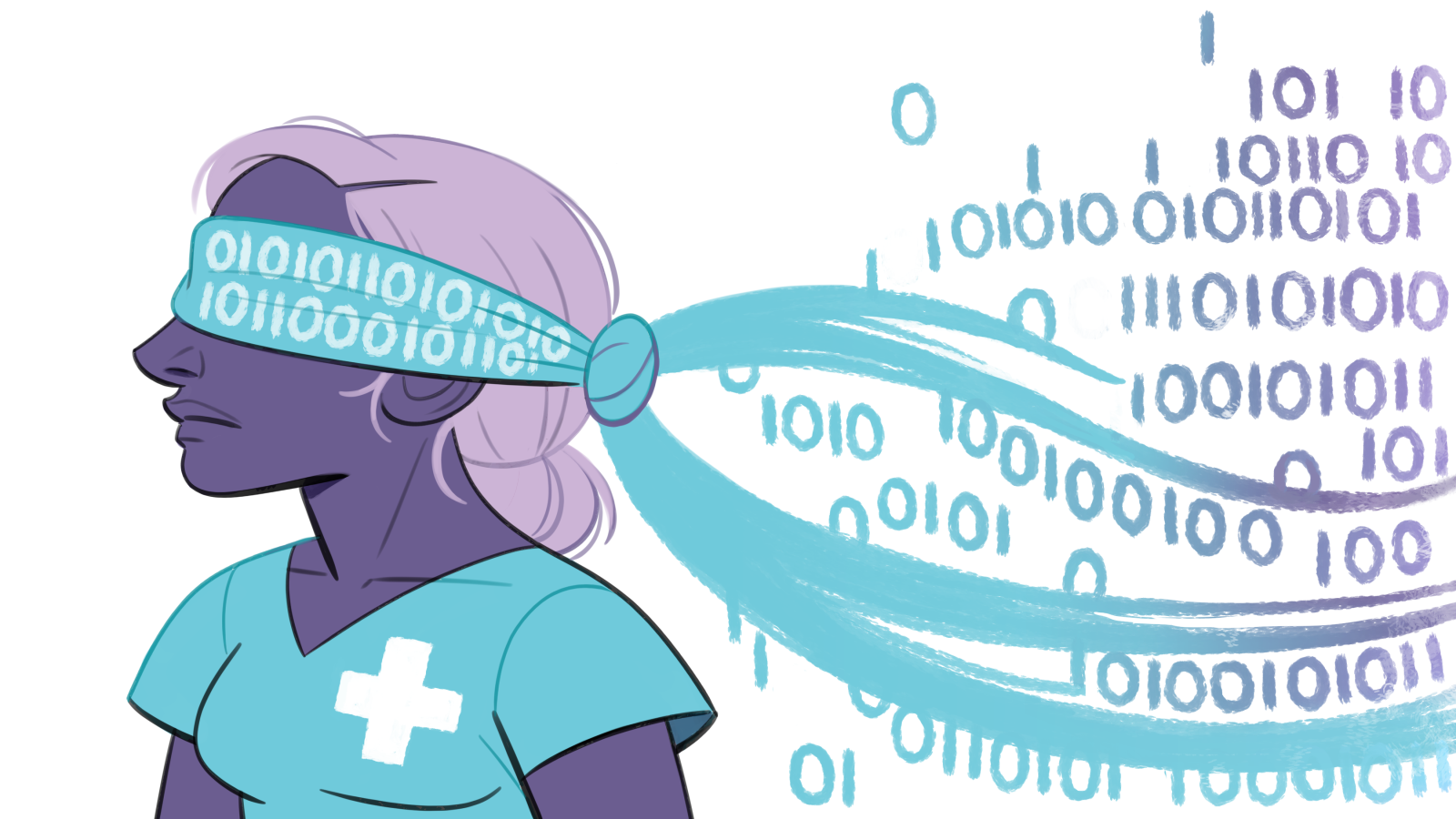
The global COVID-19 pandemic has put enormous strain on the US healthcare system. This strain has underscored racial inequity in the allocation of healthcare resources, including public health infrastructure vital to pandemic response, and in resulting health outcomes. As of December 2020, mortality rates among Black, Indigenous, and Latino Americans are estimated to be triple those of white and Asian Americans after adjusting for age.1 This stark reminder of the structural nature of racial disparity in health outcomes, alongside a national uprising led by Black people against police violence, has reenergized the movement for racial justice in the US.
Key to the demands of racial justice organizations such as the Movement for Black Lives is the redistribution of resources to make access to critical services, including healthcare, equitable.2 However, resolving inequity in healthcare will be even more complicated than enacting redistributive policy. It will also include addressing fundamental problems with the design and implementation of artificial intelligence (AI) systems that increasingly underlie healthcare decisions.
The AI systems used in healthcare are statistical models constructed using historical and demographic data and designed to produce outputs that inform decision making. Their usage in allocating resources and determining access to healthcare services has rapidly become commonplace.3 Some researchers have argued that with sufficient regulation, “[algorithms] can be a potentially positive force for equity,” by identifying and making transparent the points in a decision-making procedure where inequity may be introduced.4 It has further been argued that when bias does emerge, algorithms allow greater configurability than relying on human judgement.5
However, existing efforts to incorporate some of these considerations into the day-to-day operations of tech companies have encountered fundamental issues, calling into question whether the idea of equity-by-algorithm is practical at all.6 Recent events seem to justify this skepticism: in early December 2020, Google forced the immediate resignation of Dr. Timnit Gebru, the then technical co-lead of Google’s Ethical Artificial Intelligence team. Gebru had co-authored the well-known Gender Shades study that drew attention to racial disparity in facial recognition technology, and co-founded Black in AI. Her dismissal came after she resisted Google’s censorship of her newest research on the risks and limitations of large-scale language models, including their environmental impacts.7 Two months later, Gebru’s colleague Dr. Meg Mitchell, another Ethical AI Co-Lead at the company, was terminated after publicly criticizing Google’s actions in the lead-up and aftermath of Gebru’s departure.8
These events coincide with news that former Facebook employees departed the company over ethical concerns about the role the social network plays in political elections and platforming extremist right-wing voices.9 The willingness of companies like Google and Facebook to bet their reputations on public fights with the people who create and study their platforms should serve as a warning that similar issues are being suppressed, or more likely ignored, in more mundane settings.
While algorithms for assisting in everyday healthcare decisions typically keep a low profile, recent events — such as the deeply flawed outcome of the first scoring system for vaccine prioritization employed at Stanford Medicine, which resulted in protests by frontline healthcare workers — suggest this might not remain the case for long.10 Individual care decisions are already informed by algorithms designed by private companies seeking profit from massive healthcare IT contracts without patient consent.11
In October of 2019, Dr. Ziad Obermeyer and co-authors published an audit study of one algorithm deployed in the care of millions of US patients.12 The role of the algorithm is to automate enrollment in a high-risk health program meant to provide patients at risk for further illness with extra monitoring and preventative care. The algorithm determines a “future risk” score using a statistical model based on a detailed inventory of an individual’s characteristics, including things like past insurance claims and treatment history, but specifically excluding racial demographic information. If a patient’s score is above the 97th percentile of all scores, they will be automatically enrolled in the high-risk program; above the 55th percentile, they will be referred to their primary care physician for further consideration.
Even though the model excludes racial demographic information, the audit found that Black people who were less healthy by the metrics of the audit were assigned the same score as healthier white people, meaning that many Black patients who could benefit from enrollment in the high-risk program were left out. According to the analysis, this happened because the model used predicted healthcare costs for individual patients to decide whether they should be enrolled in the low-risk or high-risk program. Historical data used as input to the model showed lower healthcare costs for Black patients; therefore, the algorithm was less likely to suggest enrolling Black patients in the high-risk program because they appeared to be less sick from the cost-driven point of view of the model. It’s a classic example of the old computer science adage, “garbage in, garbage out” — but in this case, “garbage out” is a potentially negative health outcome for millions of people rather than a benign miscalculation or runtime error.
A wealth of social and historical scholarship exists that might have told us in advance that lower historical costs would not be a good indication of the relative health of Black people compared to whites. In a perspective on the audit study, Princeton Professor of African-American Studies Dr. Ruha Benjamin explains that the bias the authors uncover is just a new form of the same insidious problem: the structural racism of the healthcare system in the US. Benjamin emphasizes how bias issues in the algorithm are a continuation of a historical trend of disenfranchisement and maltreatment of people of color.13 Although algorithms that automate healthcare programs may not be inherently dangerous, those developed without a sociopolitical context that acknowledges and accounts for these problems in advance of deployment will always risk intensifying them. Previously published science is importantly not excluded from this sociopolitical scrutiny, as evidenced by the case of a “race correction” in testing for kidney disease based on faulty and racist science, written about elsewhere in this magazine.14
The Obermeyer study suggests addressing the bias by using a set of target outcomes rather than healthcare costs alone. The authors find that this results in a substantial reduction in bias (84% by the metrics in the study), leading them to conclude that such tweaks may remedy the issues revealed in their analysis. This solution, which assumes that the bridge between biased and unbiased algorithms is a matter of finding the right technical fixes and adjustments, follows a pattern we refer to as “careful construction.”
While Dr. Obermeyer and peers propose and analyze various tweaks that could remedy the algorithmic bias, they largely ignore the structural issues that are its source. Even assuming the tweaks are an effective way to address the specific bias in the algorithm they audited, there remains a number of other sources of bias that may influence outcomes. A separate study in 2019 led by Ninareh Mehrabi outlines 23 different kinds of bias that can affect these types of algorithms.15 The method proposed in the Obermeyer paper attempts to address issues of historical bias, referred to as “existing bias and socio-technical issues in the world,” but is unlikely to address other issues related to system-level inequities. In addition to these many individual biases, there is also the issue of evaluating the bias of algorithms in a way that accounts for the plurality and interrelation of human identities through an intersectional frame — a limitation acknowledged in the Obermeyer study. This is an idea extensively studied by socio-historical scholars, but rarely foregrounded by the designers of technology.16
As radical scientists, we know that narrow technical attempts to address bias in deployed machine learning are not enough. Careful construction misses the point by placing the focus on individual circumstances — of algorithms, of researchers, of data — rather than the systemic and structural bias inherent to algorithm design within a racist and profit-driven capitalist system. All data collection, algorithm development, and application deployment exist in a specific context that will play a role in determining outcomes. Meaningfully addressing these structural problems requires more than the careful construction paradigm alone can offer.
“Ethical AI” organizations such as the Partnership on AI have advocated for the use of algorithmic decision-making under the assumption that careful construction is possible and that it will be undertaken in the course of the rollout of such systems. However, these organizations are largely backed by major sellers of algorithms, like Google, Amazon, IBM, and Microsoft, who have supported the concept as a way of avoiding regulation while expanding the market for their tools.17 The example of Drs. Gebru and Mitchell’s exit from Google shows how these companies react when social and political issues conflict with their profit motive and the extraordinary difficulties faced by those trying to address these problems from the inside.
These circumstances, though in many ways daunting, need not immobilize us. Given the reality of continued proliferation of algorithmic decision-making across industries, we should continue the work of identifying and mitigating harms caused by these systems, while at the same time fighting for social, political, and economic systems that are oriented against injustice and exploitation. We should push any strategy for mitigating harms to include as a principle that construction of algorithmic decision-making systems must be transparently sensitive to systemic bias: not as an optional perk, but as a requirement for deployment. Further, deployment should require justification, not just based on convenience to users — an extremely low bar — but of effectiveness or potential effectiveness in improving outcomes for those whose lives may ultimately be changed by the decisions.
As it stands, “fixes” come largely in the form of unpaid collaborations between companies and universities or think tanks, the model that led to the Obermeyer audit study. These collaborations are unpaid to avoid obviously conflicting interests. Yet unless audits are truly independent, mandatory, and binding, this can mask a more subtle version of the same conflicts of interest, since the choice to continue the work is ultimately with the company providing the data rather than the researchers carrying out the audit.
There are also existing models for comprehensive regulation of technical systems that may be adaptable to AI, for example in the FDA’s methodology for assessing medical devices.18 An audit carried out in advance of deployment could have significantly altered outcomes for patients in the Obermeyer study for the better, since historical claims data — available in advance by definition — was the main input to the model. Although pre-deployment audits do nothing to fix underlying structural problems, they can identify and reduce harmful effects of bias and add evidence against the fraught claim that relying on data and models for important decisions automatically makes outcomes more fair and just.
It is vital that mitigation of harm also includes the option of foregoing algorithmic decision-making altogether, even if data and models are available. Advocates and designers of these systems will continue working to expand the applications of their technologies as they seek profits and growth. Thus in fighting algorithmic injustices we must focus not just on particular models or biases, but on the prior question of whether technology is actually a necessary or reasonable solution. This is a strategy adopted towards facial recognition technology in places like Somerville, Massachusetts, where in 2019 the city council voted to ban government use of the technology outright.19 Similar bans have been implemented in cities across the country and calls have been echoed by frontline groups seeking to abolish police use of surveillance technology, such as the Stop LAPD Spying Coalition in Los Angeles.20
The development, regulation, and deployment of algorithmic decision-making systems is rife with contradictions and complications. It is tempting to throw our hands up and either fully oppose algorithmic decision-making, or fall back into the familiar but limited careful construction approach to alleviating bias in specific circumstances with technical tweaks. However, these are not the only choices we have. By recognizing harms inherent to existing political and economic structures, and how these same structures frame the practice of science and design of technology, we can begin to address these issues in ways that are transparent and democratic. Activism, organizing, and critical scholarship on science and technology will all play important roles in this process.
This approach is a recognition of the dual nature of science, a concept coined by Dr. Richard Levins to describe the inherent contradiction of a search for objective truth within a specific social, political, and historical context that frames both the questions we ask and the potential solutions we propose.21 Rather than assuming that technical fixes will eventually solve problems of inequity, we should design our systems, and train the people who use them, to be aware of and accountable to the potential biases that exist at every step of the way. Importantly, we should also resist the temptation to pass off responsibility for important decisions, like the long-term health of our communities, to large-scale statistical models justified with the thin veneer of “scientific objectivity.”22
We should also reject the idea that issues of social, economic, and racial justice are solvable by developing and deploying the right technological systems. It is only through collective social and political action that the underlying relations that dominate society can be transformed. Any chance at a more equitable present or future in which technology plays a positive role depends on our willingness to forego profiteering in favor of a transformative, democratic approach.
—
Timothy LaRock is a scientist, organizer, and activist located in Boston, MA. He is active in the labor movement and a member of the Boston chapters of Science for the People and the Democratic Socialists of America.
Benjamin Batorsky is a data scientist located in Cambridge, MA. He is connected to various local civic technology communities and is a member of the Boston Chapter of Science for the People and the Science for the People COVID-19 response working groups.
Sam Davies is a freelance illustrator and comics artist working in the UK. She has created children’s graphic novels and is currently working on a collaboration with the University of Southampton using comics to explore autistic young people’s experiences of transitioning from primary to secondary school.
References
- American Public Media Research Lab, “The Color of Coronavirus: COVID-19 Deaths Analyzed by Race and Ethnicity in the US,” December 10, 2020, https://www.apmresearchlab.org/covid/deaths-by-race
- “End the War on Black Health and Black Disabled People,” Movement for Black Lives Policy Platform, 2020, https://m4bl.org/policy-platforms/end-the-war-black-health/.
- “Automating Society Report 2020,” Algorithm Watch & Bertelsmann Stiftung, October 2020, https://algorithmwatch.org/en/project/automating-society/.
- Jon Kleinberg et al., “Discrimination in the Age of Algorithms,” Journal of Legal Analysis, 10 (2018): 113–174, https://doi.org/10.1093/jla/laz001.
- Sendhil Mullainathan, “Biased Algorithms Are Easier to Fix Than Biased People,” The New York Times, December 6, 2019, https://www.nytimes.com/2019/12/06/business/algorithm-bias-fix.html.
- Jacob Metcalf, “Looking for Race in Tech Company Ethics,” Data & Society: Points, September 22, 2020, https://points.datasociety.net/looking-for-race-in-tech-company-ethics-956919fe48ee.
- Kim Lyons, “Timnit Gebru’s Actual Paper May Explain Why Google Ejected Her,” The Verge, December 5, 2020. https://www.theverge.com/2020/12/5/22155985/paper-timnit-gebru-fired-google-large-language-models-search-ai.
- Nico Grant, Dina Bass, and Josh Eidelson, “Google Fires Researcher Meg Mitchell, Escalating Saga,” Bloomberg, February 19, 2021. https://www.bloomberg.com/news/articles/2021-02-19/google-fires-researcher-meg-mitchell-escalating-ai-saga
- Ryan Mac and Craig Silverman, “After the Us Election, Key People Are Leaving Facebook and Torching the Company in Departure Notes,” Buzzfeed News, December 11, 2020, https://www.buzzfeednews.com/article/ryanmac/facebook-rules-hate-speech-employees-leaving.
- Caroline Chen, “Only Seven of Stanford’s First 5,000 Vaccines Were Designated for Medical Residents,” Pro Publica, December 18, 2020, https://www.propublica.org/article/only-seven-of-stanfords-first-5-000-vaccines-were-designated-for-medical-residents.
- Rebecca Robbins and Erin Brodwin, “An Invisible Hand: Patients Aren’t Being Told About the AI Systems Advising Their Care,” STAT News, https://www.statnews.com/2020/07/15/artificial-intelligence-patient-consent-hospitals/
- Ziad Obermeyer et al., “Dissecting Racial Bias in an Algorithm Used to Manage the Health of Populations,” Science 366, no. 6464 (2019): 447-453, https://10.0.4.102/science.aax2342.
- Ruha Benjamin, “Assessing Risk, Automating Racism,” Science 366, no. 6464 (2019): 421–422, http://doi.org/10.1126/science.aaz3873.
- Patricia Kullberg and Naomi Nkinsi, “How Racism Gets Baked in to Medical Decisions,” Science for the People, December 21, 2020, https://magazine.scienceforthepeople.org/web-extras/racism-medicine-naomi-nkinsi-interview.
- Ninareh Mehrabi et al., “A Survey on Bias and Fairness in Machine Learning,” arXiv (September 2019), arXiv:1908.09635 [cs.LG].
- Joy Buolamwini and Timnit Gebru, “Gender Shades: Intersectional Accuracy Disparities in Commercial Gender Classification,” Proceedings of Machine Learning Research, 81 (2018): 77–91, http://proceedings.mlr.press/v81/buolamwini18a.html.
- Rodrigo Ochigame, “The Invention of Ethical AI: How Big Tech Manipulates Academia,” The Intercept, December 20, 2019, https://theintercept.com/2019/12/20/mit-ethical-ai-artificial-intelligence/.
- “Proposed Regulatory Framework for Modifications to Artificial Intelligence/Machine Learning-Based Software as a Medical Device (SaMD),” FDA, accessed December 15, 2020, https://www.fda.gov/files/medical%20devices/published/US-FDA-Artificial-Intelligence-and-Machine-Learning-Discussion-Paper.pdf.
- Katie Lannan, “Somerville Bans Government Use of Facial Recognition Tech,” WBUR, June 28, 2019, https://www.wbur.org/bostonomix/2019/06/28/somerville-bans-government-use-of-facial-recognition-tech
- “Factsheet: Facial Recognition Technology (FRT),” Stop LAPD Spying Coalition, accessed March 8, 2021, https://stoplapdspying.org/facial-recognition-factsheet/.
- Frank Rosenthal, “The COVID-19 Pandemic and the Dual Nature of Science,” Science for the People, August 23 2020, https://magazine.scienceforthepeople.org/web-extras/covid-19-coronavirus-pandemic-science-politics/.
- Financial Times Editorial Board, “Blame Not the Robot, but the Human Behind It,” Financial Times, December 29, 2020, https://www.ft.com/content/2b7e06c2-edd0-477c-b345-45eef2851e2d