Organize the Lab: Theory and Practice
Chapter 1
Abstracting the Lab or: How I Learned to Become a Cog and Reproduce the System
By Calvin Wu
Considering how science has been practiced in the preceding four centuries, it was only a matter of time before scientists began using the revered quantitative methodology to measure their own “success.” In 2005, physicist Jorge Hirsch proposed the h-index to quantify an author’s impact.1 Since then, the use of h has become pervasive—much like that of the impact factor for scientific journals circa 1975.2 These metrics, along with other variations that serve to measure and compare, have dominated academic life. “Publish or perish,” repeats your advisor. But not only that, the publication has to make an impact. You’re climbing the academic ladder to a better place—finding a prestigious lab to do your postdoc, starting your own lab at a premier institution, getting tenure… And so, every couple of years your research productivity and impact are judged and compared with your peers.
Such a unitary prescription for scientific success (or survival) leaves very little room for anything other than selfish hoarding of merit, personal ambition, and intense competition across all spheres of academia. Pushed aside are the rationalizations for a career in science: passion for problem solving, curiosity about the world, or desire to cultivate an innate creativity. These are the contradictions you experience daily in the lab: how to practice science with the freedom it requires—not just freedom at the level of research activity and personal fulfillment, but freedom from worrying about your next evaluation, and therefore from material wants and needs.
If workers are those who must work to survive, as described by Marx in the Communist Manifesto, it is easy to see that scientists are workers too, who do not have the choice to “quit science” and live off investment income or rent. Yet, in other ways, a researcher in a lab is very different from an auto worker. Workers make goods or provide services that are sold in the market for profit. But in the lab, we make observations, generate data, and publish papers—none of these activities directly involve market exchange. This apparent distinction leads many to conclude that scientists are their own “creative” or “professional-managerial” class.3 But while scientific knowledge is not immediately for sale, the reproduction of science follows the same market logic. By situating ourselves within the process of scientific production, we may begin to identify our positions within society, thus freeing ourselves from certain illusions about the neutrality, virtue, and utility of science. More importantly, it premises our collective endeavor to change science and society.
Abstraction
The h-index may seem innocuous as an estimate or an entry to other more sophisticated methods to objectively evaluate scientific merit.4 After all, we live in the “metric society,” where every conceivable quality of social phenomena is reduced to quantities like scores, ratings, and “likes.”5 However, this overarching social practice is not an outcome of increased computational power or innovative analytics. This is a process of abstraction, the same process that has been ongoing since the dawn of modernity. As society became more dynamic through the growth of markets, general rules were needed to reduce complexity to simple concepts that confirm intuition. Yet, such an epistemological approach also influences how the society is constructed and how individuals see themselves. It is important that we follow the logic of abstraction in order to probe the inner workings of science and society: just as the value of an acre of land in sixteenth century England depended on how many sheep were reared and how much wool was sold in the Florentine market, so our value as scientists depends on inscrutable matrices of calculation that are distant to us. That h-index correlates with research funding is no more surprising than that the acreage of pasture correlates with the number of sheep.6
Is all of academic science about funding, then? Some refuse to reach such a conclusion, and some arrive by way of convoluted euphemism. In Laboratory Life (1979), sociologist Bruno Latour observed that scientists reproduce their work through the “cycle of credibility,” because scientific production is not generative without 1) money to support the lab based on a scientist’s credibility and the project’s merit, 2) credibility of data and publications that contributes to the body of scientific literature, and 3) validity and influence of research agendas and arguments that result in future research funding. Thus, credibility is what undergirds knowledge production in the lab.7 Taking Latour’s abstraction one step further, the h-index has encompassed much of the nodes in his “cycle,” from prestige, credentials, and recognition, to data and papers. However, neither Hirsch or Latour adequately accounted for funding and money.
$cience in the Lab
“Nothing so evil as money ever grew to be current among men. This lays cities low, this drives men from their homes, this trains and warps honest souls…,” proclaimed Sophocles.8 Money seemed throughout the ages antithetical to the pursuit of beauty, truth, art, and science. But even the most well-funded scientists cannot avoid some forms of accounting and budgeting in the everyday lab operation, and all principal investigators (PIs), trained in this art since time immemorial, are well-versed in the monetary anatomy of a research grant.
How each lab spends their money, of course, differs by research disciplines and problem areas; yet, all share a common statistic that 50 to 70 percent of a research grant goes to personnel cost.9 This is significantly higher than that in the business sector of a similar size venture—for example, 30 percent labor cost in a tech startup—to which a lab is often compared.10 Therefore, we can conclude that science is labor-intensive.
Moreover, if success of a lab is judged by how much funding it receives, and if funding is life-and-death for keeping the scientific enterprise afloat, however money is used, it has to be well-spent, i.e.,“returns on investment” are expected. The proposed project should materialize into high-impact discoveries that would further develop into new, fundable projects. Because research is labor-intensive, this process from one grant to another depends heavily on the productivity of lab workers. Productivity, like the h-index, is quantifiable: “how many x produced by y people in a given t.”11 In the lab, x is less concrete, but its objectification and the push for increased productivity is no less real. We have all heard, “I need this experiment (x) finished by next week (t),” “we should submit this paper (x) soon (t),” “I want (y) on this project (x) so together we can wrap up before the conference (t).”
Even without considering that the immanent drive toward productivity may lead to errors or malpractice, the money and time interaction creates additional structural effects in the lab. A project is divided and assembled through portioning the work, commonly facilitated by outsourcing and automation.12 But despite efforts from PIs and lab workers to keep the labs afloat, half of them fail within the first five to ten years.13 And for every lab that is shut down due to mismanagement or “unproductivity,” another lab grows to attain and secure a dominant position in science.14 More than just quick turnaround of results, armies of lab personnel, or efficient organization of work, what characterizes a “high-power lab” is its capacity to monopolize. It could be the mastery and development of extraordinary techniques, the ability to obtain and own sophisticated equipment or precious materials, or simply conducting research at a vastly larger scale. Not only do they require the brute force of money, it is also how the money is spent in outcompeting other labs.
All these descriptions set the operation of labs on the same structural footing as that of business enterprises. We need not rush to equate the two; it will soon be revealed that this comparison provides a powerful analytical tool, via sequential steps of abstraction, to understand society in general. For that, we need to follow the money.
Science in $ociety
In fiscal year 2022, the US federal government budgeted 171.3 billion dollars for science, and for the past decade, the top twenty US universities each have spent an average of one billion dollars a year on their research operations.15 Such large-scale scientific activity in society is relatively recent. For most parts of the history of Western science since the fifteenth century, the money for science came from patronage.16 Even in Victorian Britain, at the height of the second industrial revolution and British global hegemony, Parliament only allotted meager sums for its Royal Society.17 It was only after the Second World War that resources were mobilized by the state for scientific research.18 Thus, the justification for science funding is not simply about knowledge or progress, because history has seen groundbreaking inventions and theoretical advances without government-funded research projects.
We can obtain sociological answers from a wealth of literature about the social function of science, or we can follow the abstraction already made by policy makers. Here I present an example from an analysis of Sweden’s research and development goals.19 Short of being called an index, the below framework reduced the function of science research in society into a scorable matrix:
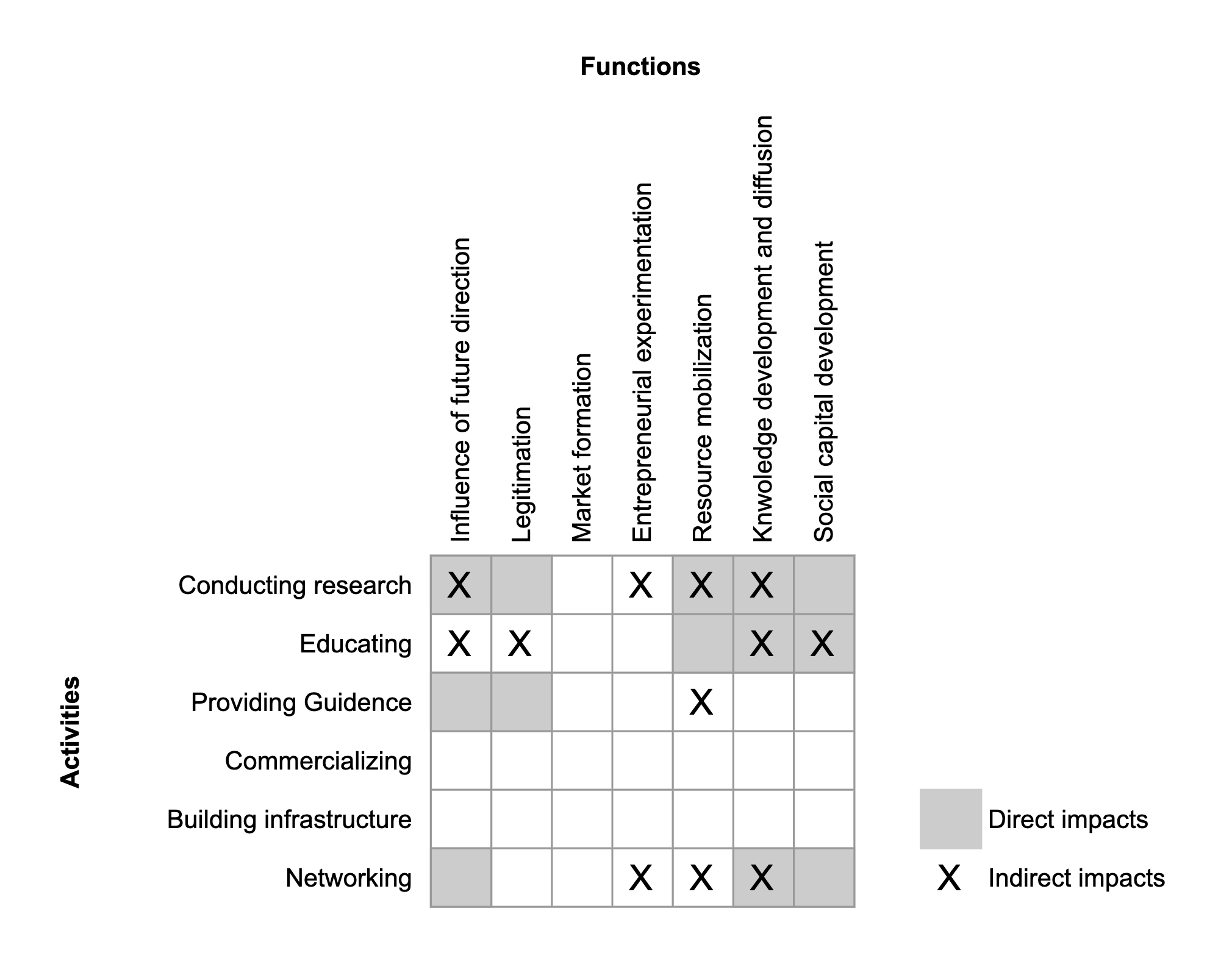
This framework attempted to expand the narrow criteria of judging the success of science by the number of publications or whether it leads to patents for commercialization. But similar to Latour’s “cycle of credibility” at the ethnological level, “entrepreneurial experimentation,” “market formation,” and “resource mobilization,” are just different aspects of the monetary calculation. Interestingly, the other categories, “influence of future direction,” “legitimation (acceptance by the public),” “knowledge,” and “social capital development,” reflect the reproduction of labor (focus of the next section).20 What is preeminent here is that the labs are, in fact, linked to the market and that scientists actually do move money around.
The government not only funds labs; for every dollar a lab receives in external funding, the institution where the lab is housed receives an additional 50 to 90 cents.21 The rate is negotiated by the institution, which has complete discretion over usage of these funds; some would be used to support research facilities, administrations, and personnel costs, and some may be pooled into their growing investment funds. These are huge sums of money.22 The University of Michigan, for example, is the fifth largest employer in the state and touts an endowment fund of $17 billion (for perspective, Ford Motor Company’s endowment is $16 billion). Columbia University, in addition to its endowment, also holds commercial land holding as the seventh largest real estate owner in New York City.23 These institutions, thus, can exert tremendous economic power.24
So, we scientists push money from the government to the university, while impacting future movement of money, shaping public views on research, testing infrastructure for future investments, and creating new markets. When it comes to money, it becomes obvious why competitions within and among labs are rampant, why time and productivity pressurize lab workers, why operations in labs are often hierarchical, and why one does not feel free in the lab.
Ideological Scientific Apparatuses
Being shackled to the larger economic structure immediately reveals some of science’s more nefarious aspects: for example, military research—innovations of destruction—as the birth pang of American scientific boom, the fact that eugenicist research continues to this day, and how the privatization of public-funded research leads to vaccine apartheid.25 Less conspicuous, however, is science’s role in reproducing our current social system. Let us loop back to the recurring idea from Latour and from the research impact matrix about “knowledge.” Universities are more than business enterprises; they actually provide education and transfer knowledge!
Just like individual achievement or social impact, knowledge and education can also be abstracted. Standardized testing and grade-point averages reduce education and knowledge to measurable quantities in the same way as the h-index for a scientist’s work. And again, abstraction allows us to see the movement of something; in this case, it is not money, but labor. Remember, science is labor-intensive; new workers need to be trained, first by the larger educational system, then by the research institutions, then by the academic ladder itself. This cycle of reproduction splits the lab into distinct functional compartments—teaching and research, training and production, mentorship and entrepreneurship—that increasingly polarize workers within and present scientists with irresolvable contradictions.
To be trained to reproduce the lab through recycling money and labor is to become a “one-dimensional” scientist.26 The content of scientific knowledge necessarily conforms with the modus operandi of the abstracted scientific enterprise. Consider the kind of knowledge generated by the ever finer divisions of labor. Increasingly specialized, under the productivity regime, new lab trainees see the world with ever narrower scopes. There is no time for scientists to ruminate over the historical trajectory, social development, and political implications of their research. In place of the broader understanding as elucidated so far in this article, a reified consciousness of scientism takes over. “Appreciating nature,” “understanding human existence,” or “achieving progress,” these romanticized notions are not specious per se, but they serve to mystify science from even scientists themselves, and provide vested interests with the justification for cost, benefit, and externalities of science. This underlies the concept of “ideology,” which coerces individuals, doubly by force (threat of unemployment) and by manufactured consent (transfering of specific knowledge through education), to reproduce the system.27
In reiterating that science is political, we have taken a first step in unveiling the role of science in society. Next we must ask: what are our positions within?
€xploitation
We have so far steered away from making distinctions among the different roles in the lab and treated the lab as a homogenous unit in processing money and knowledge. There is a certain degree of autonomy for individual labs as to how they are organized. Many labs do function like small businesses, with the PI as the “owner-manager” at the top of the hierarchy and with multiple ranks sorted by career levels. Yet, other labs can also take a more horizontal and cooperative organization. The lab’s budget and problem areas can be dictated by a single person on top, or they can be democratically and collectively decided. What I argue here is that it is not so much the power relations within the lab—which may or may not reflect power relations on the social scale—but the economic forces that define our class identity.
Not unlike workers in a traditional sense who produce goods and services on the market, the scientist’s work is also integrated into a social circuit, heavily influenced, if not all mediated by the same monetary calculation. While observations, data, papers are not for sale, their production pushes money around, enriches institutions, maintains social reproduction, and creates new spheres for investment. Marx defined the working class by the fact that through working, workers reproduce their own situation as workers, that they have no escape, as working is their only way to survive society. Therefore, class should be seen as an ongoing process in relationship to another.28 For example, in the seventeenth century, artisans did not see themselves as workers; it was only since the nineteenth century with intensified competition from industrial production that they understood the process of class polarization. Similarly, scientists first came from the aristocracy, then from the wealthy middle-class urban lots, and then from the working class.
Yet, the formal difference between lab work and factory work has an effect on scientists’ working-class consciousness. The geographical, juristical, and pecuniary differences among academic institutions lead scientists to perceive differently their precarity. Across different scientific disciplines, each scientist at different career levels may question whether they actually have “no choice” but to work. Stories about scientists living off intellectual patent rights or venture capital are repeated—the fact that they are extremely rare yet extremely well-known should tell us something about ideology.
Related to perception, everyday experience in the lab also influences how scientists understand exploitation.29 The dual function of labs as sites of education and of production complicates the matter. Workers and owners are antagonistic; trainees and mentors are not. Moreover, just as lab organizations are heterogenous—hierarchical vs. cooperative, pyramidal vs. horizontal—so the PI’s roles are amorphous. In conventional enterprises, managers align with the owner’s interest; in this sense, it is not apparent that PIs necessarily have the same interest as university regents. Yet, despite the lab’s autonomy, PIs are not owners, because what they “own” has no value outside of the established structure of an academic institution. An important definition of being an owner is that they possess the choice to close shop and live off money—the choice not available to workers—a scientist, no matter how prominent, cannot live off h-index alone.
Finally, what a factory or service worker generates for the boss is rather transparent; their leverage as workers in collective struggle—the ability to withhold labor—directly translates to the absence of profit, a quantifiable amount. Setting political matters aside, the eternal push and pull in labor negotiation begins with a series of financial accounting: how much profit is lost if or when how many workers go on strike and for how long, and how much would it cost to replace workers respective of the said strike. But the differential equation between scientific labor and profit is not linear. Similarly, our leverage as lab workers also differs from that of the skilled trade model of organizing, which hinges on the relative monopoly of expert knowledge and the management’s inability or unwillingness to replace workers. Scientific “skills” are hyper-specialized, lab-specific, and difficult to generalize in collective bargaining. Amassing worker’s leverage across labs thus requires further strategic considerations.
—
It must be emphasized that the reduction of science to economic activity is not the conclusion but rather the premise of this analysis. If the International Monetary Fund and the World Bank both put a price on lab research, who are we to deviate from the ruling class orthodoxy?30 It is not to discard the qualitative content of science by quantitative abstraction. After all, scientific advancement in the past few hundred years contributed greatly to human’s understanding and interaction with nature. Yet, just as Marx admired modernity for its “wonders far surpassing Egyptian pyramids, Roman aqueducts, and Gothic cathedrals,” so he forewarned about its “[converting] the man of science into paid wage laborer.”31 The contradictions in everyday lab life are thus laid bare. The struggle to overcome precarity and the process of scientific production are intricately linked. The forcing of lab workers to work longer hours, the low pay and high living expense, the lack of benefit and security on the one side, and the narrowing of creative freedom, crumbled methodological integrity, unfree exchange, and dubious social utility—the warping of honest souls—on the other, are mutually reinforcing under the structure and function of academic science, and writ large, our capitalist society.
Back to Contents
Notes
- J. E. Hirsch, “An Index to Quantify an Individual’s Scientific Research Output,” Proceedings of the National Academy of Sciences of the United States of America 102, no. 46 (November 15, 2005): 16569–72, https://doi.org/10.1073/pnas.0507655102.
- Paul Wouters, “Eugene Garfield (1925-2017),” Nature 543, no. 7646 (March 22, 2017): 492, https://doi.org/10.1038/543492a.
- Alex Press, “On the Origins of the Professional-Managerial Class: An Interview with Barbara Ehrenreich,” Dissent, October 22, 2019, https://www.dissentmagazine.org/online_articles/on-the-origins-of-the-professional-managerial-class-an-interview-with-barbara-ehrenreich; Richard Florida, “Maps Reveal Where the Creative Class Is Growing,” Bloomberg News, July 9, 2019, https://www.bloomberg.com/news/articles/2019-07-09/maps-reveal-where-the-creative-class-is-growing.
- Those arguing against the use of h-index are oftentimes trying to come up with yet another metric. See Vladlen Koltun and David Hafner, “The H-Index Is No Longer an Effective Correlate of Scientific Reputation,” PloS One 16, no. 6 (June 28, 2021): e0253397, https://doi.org/10.1371/journal.pone.0253397.
- See Steffen Mau, The Metric Society: On the Quantification of the Social (John Wiley & Sons, 2019).
- Smita Saraykar, Ayman Saleh, and Salih Selek, “The Association Between NIMH Funding and H-Index in Psychiatry,” Academic Psychiatry: The Journal of the American Association of Directors of Psychiatric Residency Training and the Association for Academic Psychiatry 41, no. 4 (August 2017): 455–59, https://doi.org/10.1007/s40596-016-0654-4; P. S. Pagel and J. A. Hudetz, “H-Index Is a Sensitive Indicator of Academic Activity in Highly Productive Anaesthesiologists: Results of a Bibliometric Analysis,” Acta Anaesthesiologica Scandinavica 55, no. 9 (October 2011): 1085–89, https://doi.org/10.1111/j.1399-6576.2011.02508.x; Issa Rezek, Robert J. McDonald, and David F. Kallmes, “Is the H-Index Predictive of Greater NIH Funding Success among Academic Radiologists?,” Academic Radiology 18, no. 11 (November 2011): 1337–40, https://doi.org/10.1016/j.acra.2011.06.017.
- Bruno Latour and Steve Woolgar, Laboratory Life: The Construction of Scientific Facts (Princeton University Press, 2013): 201.
- Sophocles, Antigone (New York: Dover Publications, 1993).
- For an example of a research budget, see “Grant Budget Calculation Information,” University of Florida, accessed June 5, 2022, https://psych.ufl.edu/info-forms/grants/psychology-grant-budget-calculation-information/; “Create a Budget,” National Institutes of Health, accessed July 2, 2022, https://www.niaid.nih.gov/grants-contracts/create-budget.
- Wasim Jabbar, “How Much Money Does A Tech Startup Need?,” Business Data List, April 11, 2022 https://businessdatalist.com/how-much-money-does-a-tech-startup-need/.
- Giovanni Abramo and Ciriaco Andrea D’Angelo, “How Do You Define and Measure Research Productivity?,” Scientometrics 101, no. 2 (November 1, 2014): 1129–44, https://doi.org/10.1007/s11192-014-1269-8.
- Erika Check Hayden, “The Automated Lab,” Nature 516, no. 7529 (December 4, 2014): 131–32, https://doi.org/10.1038/516131a; Beryl Lieff Benderly, “Outsourcing, Coming Soon to a Lab Near You,” Science, September 7, 2016, https://doi.org/10.1126/science.caredit.a1600127; James Boyd, “Robotic Laboratory Automation,” Science 295, no. 5554 (2002): 517-8, https://doi.org/10.1126/science.295.5554.517; Sarah Moore, “Future of Lab Automation,” AZO Life Sciences, March 20, 2022, https://www.azolifesciences.com/article/Future-of-Lab-Automation.aspx.
- Sally Rockey, “Retention Rates for First-Time R01 Awardees,” NIH extramural NEXUS, October 28, 2014, https://nexus.od.nih.gov/all/2014/10/28/retention-of-first-time-r01-awardees/.
- The “Matthew effect,” or “success begetting success,” has been empirically and quantitatively demonstrated for STEM academia. See Alexander M. Petersen et al., “Quantitative and Empirical Demonstration of the Matthew Effect in a Study of Career Longevity,” Proceedings of the National Academy of Sciences of the United States of America 108, no. 1 (January 4, 2011): 18–23, https://doi.org/10.1073/pnas.1016733108; Thijs Bol, Mathijs de Vaan, and Arnout van de Rijt, “The Matthew Effect in Science Funding,” Proceedings of the National Academy of Sciences of the United States of America 115, no. 19 (May 8, 2018): 4887–90, https://doi.org/10.1073/pnas.1719557115.
- U.S. Library of Congress, Congressional Research Service, Federal Research and Development (R&D) Funding: FY2022, by John F. Sargent Jr., R46869(2022), 3, https://sgp.fas.org/crs/misc/R46869.pdf; “Ranking by Total R&D Expenditures,” National Science Foundation, accessed April 1, 2022, https://ncsesdata.nsf.gov/profiles/site?method=rankingbysource&ds=herd.
- Vera Keller, “Before Nobels: Gifts to and from Rich Patrons Were Early Science’s Currency,” The Conversation, October 4, 2016, http://theconversation.com/before-nobels-gifts-to-and-from-rich-patrons-were-early-sciences-currency-66360.
- Roy M. Macleod, “The Support of Victorian Science: The Endowment of Research Movement in Great Britain, 1868–1900,” Minerva, 1971, https://www.jstor.org/stable/41822100.
- It is not a coincidence that the Second World War is a watershed moment in science funding. The post-War economic boom—and in effect, scientific boom—is intricately linked to military expenditure and the economic system of large-scale production and consumption. See Calvin Wu, “Socialize the Lab,” Science for the People, September 15, 2021, https://magazine.scienceforthepeople.org/online/socialize-the-lab/.
- Staffan Jacobsson and Eugenia Perez Vico, “Towards a Systemic Framework for Capturing and Explaining the Effects of Academic R&D,” Technology Analysis & Strategic Management 22, no. 7 (October 1, 2010): 765–87, https://doi.org/10.1080/09537325.2010.511140; Staffan Jacobsson, Eugenia Perez Vico, and Hans Hellsmark, “The Many Ways of Academic Researchers: How Is Science Made Useful?,” Science & Public Policy 41, no. 5 (January 3, 2014): 641–57, https://doi.org/10.1093/scipol/sct088.
- “Social capital development” is essentially the upkeep of the labor market that may be tapped by future investment.
- Heidi Ledford, “Indirect Costs: Keeping the Lights on,” Nature 515, no. 7527 (November 20, 2014): 326–29, https://doi.org/10.1038/515326a; “Indirect Cost Rate Survey,” Datahound, May 10, 2014, https://datahound.scientopia.org/2014/05/10/indirect-cost-rate-survey/.
- “Endowments,” Fast Facts, National Center for Educational Statistics, accessed April 1, 2022, https://nces.ed.gov/fastfacts/display.asp?id=73.
- “Ivy League Universities or Real Estate Kings?,” Reonomy, May 1, 2020, https://www.reonomy.com/blog/post/ivy-league-universities-or-real-estate-kings.
- With money at stake, it becomes apparent why fossil-fuel divestment campaigns are moving at a glacial pace.
- Ed Romano and Grace Huckins, “Racism and Repair: Science Under White Supremacy,” Science for the People 23, no. 3 (Winter 2020): 65–69, https://magazine.scienceforthepeople.org/vol23-3-bio-politics/racism-repair-science-white-supremacy/; Clifford D. Conner, The Tragedy of American Science: From Truman to Trump (New York: Haymarket, 2020); Winnie Byanyima, “A Global Vaccine Apartheid is Unfolding. People’s Lives Must Come Before Profit,” The Guardian, January 29, 2021, https://www.theguardian.com/global-development/2021/jan/29/a-global-vaccine-apartheid-is-unfolding-peoples-lives-must-come-before-profit.
- A “one-dimensional man” is an individual integrated into the capitalist society under various forms of social control, resulting in conformity of thoughts and behaviors. See Herbert Marcuse, One-Dimensional Man: Studies in the Ideology of Advanced Industrial Society (Boston: Beacon Press, 1964).
- Valeriano Ramos, Jr., “The Concepts of Ideology, Hegemony, and Organic Intellectuals in Gramsci’s Marxism,” Theoretical Review 27 (March–April 1982), https://www.marxists.org/history/erol/periodicals/theoretical-review/1982301.htm.
- Karl Marx, Capital: Volume One (New York: Penguin Books, 1976), 799.
- Here, exploitation is used in psychological and not economic terms.
- World Bank, “Innovating through Science and Technology,” World Development Report 2008: Agriculture for Development (October 2007), https://elibrary.worldbank.org/doi/abs/10.1596/978-0-8213-6807-7_ch7; Philip Barrett et al.,“Why Basic Science Matters for Economic Growth,” IMF Blog, October 6, 2021, https://blogs.imf.org/2021/10/06/why-basic-science-matters-for-economic-growth/.
- Karl Marx and Frederick Engels, The Communist Manifesto (New York: International Publishers, 1948), 11.