March 29, 2021
The Cracked Crystal Ball of COVID Models
By Jacklin Kwan
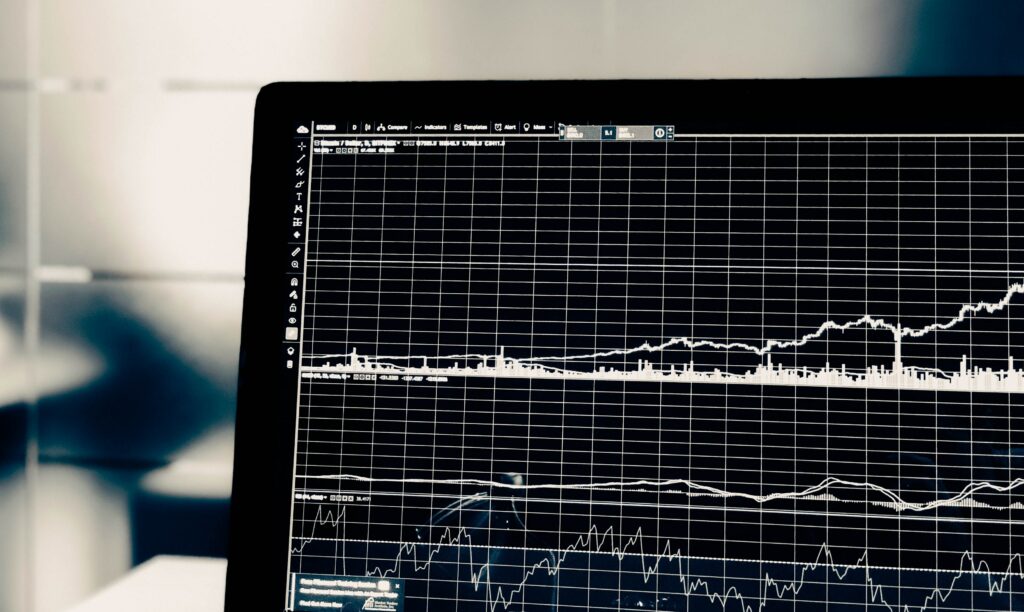
More than a year after COVID-19 was first reported to the World Health Organization, the decade-defining pandemic has revealed deep structural flaws in the policy-making, scientific communication, and economic resilience of many nations. But while the pandemic is a deeply complex and emotional tragedy for many individuals, much of it has been seen through the lens of corporate structures that promise simplicity and control through pragmatic risk assessments — the kingpin of which is modeling and forecasting.
Modeling is founded on the idea that we are exposed to a host of risks that can be transformed into quantifiable and manageable variables like projected revenue losses, probabilities, or insurance costs. The need for the security of such numbers has intensified during COVID-19; they are appealing to those who wish to answer questions such as where outbreaks will next occur, which supply lines the outbreaks will disrupt, and how infection rates are likely to grow in different situations. It is not at all surprising that many consulting firms have leaped at the opportunity to create their own models, available for the right price. Indeed, models lend security and certainty to an uncertain world, but there exist dangers of them being applied opaquely, erratically, and irresponsibly to justify policy actions that harm people.
In a pandemic that could last for many months, epidemiological models are prone to major inaccuracies. It is crucial to acknowledge these inaccuracies when applying the models responsibly, but they are rarely revealed to the public. The lack of transparency around the technology of modeling has meant the rise of two pernicious effects. First, contractors who model the effects of COVID-19 can market false confidence to their clients and undersell the presence of uncertainties. Second, companies can use the pseudo-objectivity of models to justify drastic corporate decisions, such as mass lay-offs.
Problems with model-based decision-making in the UK occurred as early as April, one month after the start of the country’s first wave of infections. Between April and May 2019, large UK universities began to announce a significant wave of wage cuts and large-scale terminations for staff1. Many universities conducted internal risk assessments which predicted a dramatic decrease in student income. Some were based on surveys conducted by Quacquarelli Symonds and the British Council. These surveys asked students about their intentions to attend or continue their university education at a time when many were unsure of their prospects. Forecasts based on these survey results predicted that the global market for international students would be depressed by more than 50 per cent for the next few years. In light of these predictions, universities in Bristol, Edinburgh, Newcastle, Sussex and Manchester jumped to cut costs. The University of Manchester specifically defended that this 50 percent reduction — equaling a loss of £270 million — was within the bounds of prudent modeling.
It wasn’t long before the University College Union (UCU), a trade union of over 130,000 university staff across the UK, challenged such conclusions. They commissioned a report by London Economics which predicted much smaller losses, averaging £20 million per institution, with the Russell Group, an association of the UK’s leading research universities, losing around £50 million2. And unlike many universities, London Economics published the methods and data sets behind their calculations so that their results could be replicated.
Even with groups like London Economics challenging the conclusions that institutions made in their modeling, reactionary cost-cutting measures undertaken by universities continue to impact their staff. More than six months into the pandemic, job security for many academics on fixed-term contracts has been drastically reduced despite avoiding the steep shortfall of students that many administrators predicted in the first academic semester3.
Since most people understand models to be constructed from objective scientific facts, the decisions made from their predictions are therefore presumed to be rational, and legitimate. But different models lead to wildly different conclusions, and most often only the most powerful voices control the decisions. The UCU were correctly skeptical about the assessments that universities made, and the most precarious workers were made to bear the brunt of those assessments. The economically vulnerable — in this case, academic staff on fixed-term contracts — have risked large-scale redundancies and wage cuts in a misguided effort to cut costs when powerful models predicted the worst.
Often, business consultants use models to present a best-case and worst-case scenario to their clients. Any decision taken from that stage onwards is an inherently political one about the type and quantity of risk the client is willing to accept. This ‘risk appetite’ is a value judgement on how much risk is acceptable, what trade-offs a group is willing to make, and who they are willing to place the burden of negative consequences on. These concerns are not and should not be portrayed as matters of scientific fact.
To properly critique models in practice, we must first understand how they operate.
There are two main types of models: 1) short-term statistical predictions that operate over just a few weeks at most, and 2) mechanistic models that investigate possible scenarios. They serve different functions and hence have different limitations and strengths.
Short-term statistical predictions use existing data to predict the next term in a sequence of numbers, and can be highly accurate in certain contexts. For example, linear regression works by fitting a line to two-dimensional data. Machine learning, on the other hand, employs artificial intelligence to learn from available data sets and discover patterns hidden in the data to make predictions. For example, based on recent trends in infection rates, it can predict future rates by extrapolating the trend into the future. This does not rely on the designer having to consider the mechanisms of virus transmission. They are often used to produce quantitative predictions for policymakers, but their accuracy drops steeply over time, often within the span of a few weeks4.
Mechanistic models, on the other hand, are used to simulate future scenarios. Though the models being developed by groups are unique, the mathematical principles that underpin them are similar. The simplest ones consist of generalizations about how a population becomes susceptible to the virus, then become infected, and then either recover or die– known as ‘S-I-R models.’5 The models are fitted and tested against some chosen set of past data. Mechanistic models are often used to formulate long-term strategies such as vaccine dispensing, travel restrictions, or shelter-in-place orders.
The distinction between short-term and long-term predictions is exemplified in weather forecasting. We can predict tomorrow’s weather to a reasonably high degree of accuracy, but it would be impossible for meteorologists to predict the weather three months from now to the same degree of accuracy. But while short-term forecasting fails for the far future, we can apply long-term predictions for other atmospheric trends, like global temperature increases, using environmental variables that are more easily modeled or simulated. Similarly, mechanistic models can often predict more generalized and averaged trends in, say, national or regional infection rates. Scientists often use both to fully inform medical policy: short-term decisions about resource allocation are made using statistical models, while mechanistic models are meant to test large-scale lockdown scenarios and vaccine rollouts.
When conveyed like this, it seems deceptively simple. The reality is anything but. Unlike weather and climate datasets that have been collected over decades, COVID-19 datasets are smaller, and of poorer quality6. The lack of robust and widespread virological testing means that the number of cases is underestimated. Even after a year since the first case was detected in Wuhan, there is relatively little data, so large sample sizes that can represent populations of interest may be difficult to find. Small sample sizes also lead to an increased risk of overfitting, when a statistical model begins seeing patterns in randomness rather than detecting a real relationship between different variables. Especially if more complex modeling strategies are used, fewer data points means a greater risk of finding false patterns. Fitting and testing models to these incomplete data sets may significantly reduce their accuracy. Additionally, researchers’ own biases often come into play when selecting which data sets to use in fitting and testing their model7
Furthermore, many aspects of virus transmission are still poorly understood, such as how biological features of COVID-19 impact how the virus is transmitted. Many simplifications about virus transmission have to be made, including ones about individual social behavior, like how many people one person interacts with, or what high-risk activities they may engage in.
Due to these factors, many models may have high degrees of inaccuracy and uncertainty. Moreover, this uncertainty is often calculated or recorded in a non-standardized way8. Profit-driven firms that create in-house models may actually benefit from the lack of an industry or academic standard as it allows them to more easily understate biases, inaccuracies and errors in their product.
Even if confidence intervals-a range of plausible values used to convey the certainty of a measured parameter-accompany epidemiological results, these may not fully capture the uncertainties associated with the data and model assumptions. Underestimating these uncertainties provides consumers a heightened sense of false confidence since they cannot easily critically engage with the model’s methodology or compare its performance to a high-quality benchmark. More cynically, corporate consumers may understand the presence of uncertainties and errors, but may intentionally underplay their importance if they have perverse incentives to pursue a certain policy decision. Decisions made based on these epidemiological outcomes require many value judgements that are far from straightforward.
More needs to be done to ensure that a variety of stakeholders are consulted throughout the risk assessment process, and that the methodologies and data sets behind the bottom-line calculations are made transparent to third-parties. Public understanding about the inherent limitations of COVID-19 models must be enhanced if workers and voters are to challenge the damaging decisions taken by corporations or politicians. People must understand that COVID-19 forecasts are not the end-all and be-all of decision-making during the pandemic. Learning to question the data analyses that underpin models, as well as dismantling their facade of objectivity, will be powerful tools in the next few months to ensure workers’ rights and voters’ interests.
—
Jacklin Kwan is a journalist based in Manchester, United Kingdom. She has written for Wired UK, Container Magazine, and Footprint Magazine on science, emerging technologies, and the environment.
References
- Madeline Bodin, “University Redundancies, Furloughs and Pay Cuts Might Loom amid the Pandemic, Survey Finds,” Nature, July 30, 2020, https://www.nature.com/articles/d41586-020-02265-w.
- Maike Halterbeck et al., Impact of the Covid-19 Pandemic on University Finances (London: London Economics, 2020), 13-14, https://londoneconomics.co.uk/blog/publication/impact-of-the-covid-19-pandemic-on-university-finances-april-2020/.
- UCAS. 2020 End of Cycle Report (Cheltenham: UCAS, 2020).
- Nicholas P. Jewell, Joseph A. Lewnard, and Britta L. Jewell, “Predictive Mathematical Models of the COVID-19 Pandemic,” Journal of the American Medical Association 323, no. 19 (April 2020): 893, https://doi.org/10.1001/jama.2020.6585.
- Neil M Ferguson et al. “Impact of non-pharmaceutical interventions (NPIs) to reduce COVID-19 mortality and healthcare demand,” Imperial College London (London: Imperial College London, 2020), https://doi.org/10.25561/77482.
- Hamid R. Tizhoosh and Jennifer Fratesi, “COVID-19, AI Enthusiasts, and Toy Datasets: Radiology without Radiologists,” European Radiology (Nov 2020): 1-2, https://doi.org/10.1007/s00330-020-07453-w.
- Eliane Röösli, Brian Rice, and Tina Hernandez-Boussard, “Bias at Warp Speed: How AI May Contribute to the Disparities Gap in the Time of COVID-19,” Journal of the American Medical Informatics Association 28, no. 1 (August 2020): 190-192, https://doi.org/10.1093/jamia/ocaa210.
- Inga Holmdahl and Caroline Buckee. “Wrong but Useful — What Covid-19 Epidemiologic Models Can and Cannot Tell Us,” New England Journal of Medicine 383, no. 4 (July 2020): 303-305, https://doi.org/10.1056/NEJMp2016822.